Estimated reading time: 6 minutes
A common challenge for healthcare systems is how to properly segment their patient populations based on risk profiles and comorbidities. When done well, segmentation ensures a high quality of care delivery and superior patient outcomes, all while minimizing risks of fines and penalties for the health system. Tactically however, this is difficult to do, especially when further exacerbated by high-risk patients being admitted for in-patient treatment in its facilities. While stratifying patients based on their 30-60-90 day readmission risk is daunting for health systems, it’s mission-critical for all involved. Let’s take a closer look at how health systems using augmented analytics can meet this challenge and thrive.
First, health systems need a holistic approach to address these challenges. The need to segment patient populations must come from a strategic perspective, and then tactically stratify them based on risk to ensure superior patient outcomes at a lower cost of care delivery. This will help to minimize risk of fines and penalties associated with readmissions and non-compliance.
Here is a best practice model that has been adopted by leading health systems as shown below:
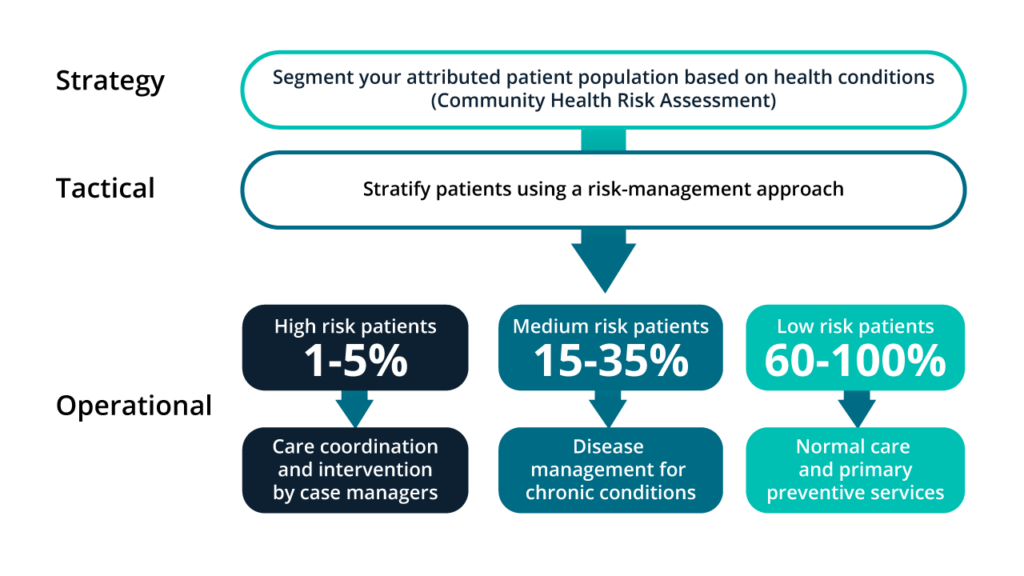
Let’s look at how this works, specifically how to approach segmentation from strategic, tactical and operational views.
Strategic: Population health segmentation and community health assessments are critical to assess the state of health of the population being served. A community health assessment refers to the process of community engagement, collection, analysis and interpretation of data on health determinants, health outcomes, and health disparities--as well as identification of resources to fulfill these needs and ensure superior patient and population health outcomes. The CDC has pinpointed and articulated 42 metrics for health determinants and health outcomes that, if measured and analyzed correctly, will provide healthcare providers with an accurate blueprint of the health of the population being served. These can then be leveraged to segment the population, based on risk and cost to serve, to drive a pragmatic population health management strategy. This allows providers to deliver the highest quality of care cost effectively while managing risk.
Tactical: Patient risk stratification using a risk-based management approach: This is arguably one of the most challenging aspects of population health management. It demands sophisticated machine learning and advanced predictive analytics software. And, that software must leverage complex models to predict risk at both an aggregate population level and a discrete patient level. Leveraging solutions like JVION, industry leaders are stratifying their patients based on 30-day readmission rate risk, risk of overshooting their length of stay, and other key performance indicators into high-risk patients. This includes multi-morbid, catastrophic conditions (e.g., heart attacks, heart failure, cancer) medium-risk, chronic conditions (e.g., diabetes, arthritis, Alzheimer’s, Parkinson's), and low-risk, preventable conditions.
Operational: Risk-based treatment and case management for high-risk patients involves care coordination, intervention by nurse case managers, and a care ecosystem comprising family, friends and social workers. For medium-risk patients, the plan would include enrollment to a health plan-funded wellness and disease management program for effectively navigating chronic conditions. Treatment of low-risk patients would be ‘business as usual’ with primary care and preventive services.
Augmented analytics is the solution:
The approach identified above is daunting to address from a healthcare IT and analytics perspective for the following reasons:
- The relevant patient data is stored in multiple, often disconnected systems and is difficult and expensive to integrate.
- KPIs and metrics for risk stratification often involve prepping, blending and normalizing disparate data elements to ensure quality and accuracy of these metrics.
- Addressing the first two considerations often results in a rear-view mirror snapshot. Basically, you’re looking at “what has happened” (descriptive analytics) instead of “why did it happen” (diagnostic analytics) or more importantly, “what will happen” (predictive analytics).
Our approach to augmented analytics
Risk stratifying patients is essential to managing gaps in care, ensuring superior patient outcomes and maintaining compliance. Augmented analytics critical to addressing and resolving the challenges that get in the way of stratification success--all the while boosting staff productivity and efficiency.
In our attempts to drive to superior patient outcomes, we want to consider how we review their care path. We want to focus on and prioritize measures that will help healthcare providers and clinicians deliver high quality, affordable health care for our patients. To meet this goal, we need to do it consistently and also scale to meet the demands of our patient populations. You need actionable insights that are grounded on market-based clinical, cost and utilization standards and best practices. You want to consider methodologies that can enable benchmarking across patient populations such as risk, SDOH, gender, age, geography, comorbidities, etc. Building all these tools and capabilities from scratch is a huge and costly venture, but having all these capabilities pre-built and delivered out-of-the-box (or with easy configurability) greatly accelerates the time-to-value and impact.
Business needs are moving at ever-increasing speeds. We are dedicated to ensuring rapid turnaround times, meaning you can access actionable insights “at the speed of business.” And, we want to do that in a way that facilitates exploration of data with ease to understand where the most impactful opportunities lie. Here are a few examples:
Examine patient risk with Care Gap Search
Quickly and easily identify patients with specific chronic conditions and gaps in care. This enables the user to search for specific patients to understand their current gaps in care, allowing for appropriate and timely intervention to manage patients into compliance.
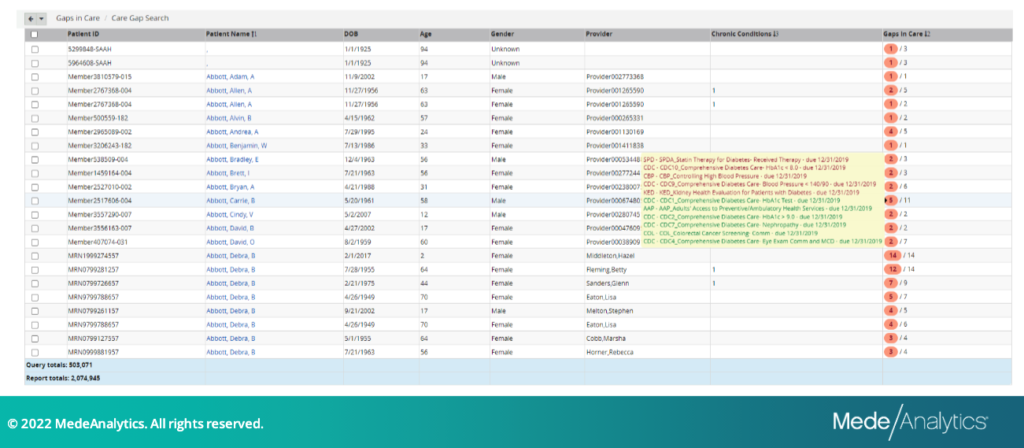
Reduce time spent interpreting reports with key metrics displayed and explained
The program instantly drives you to insights such as the largest value or greatest increase/decrease of a value in a narrative that is contextual to the data represented.
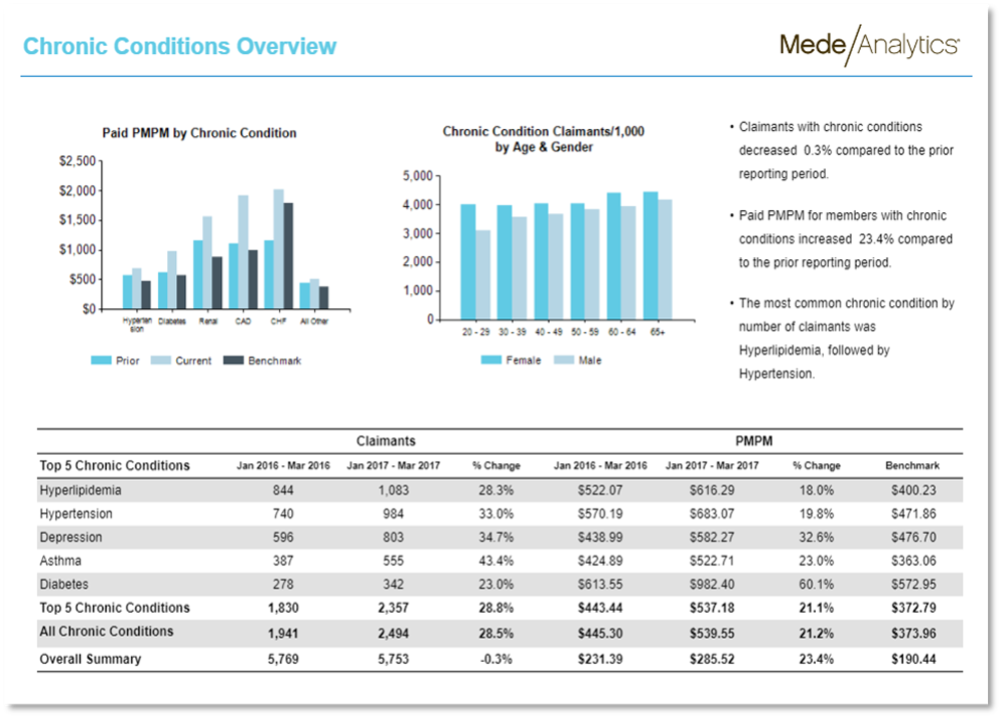
Let’s take a look at a real-world example:
A risk-bearing ‘payvider’ for Medicare and Medicaid patients leveraged predictive analytics from MedeAnalytics to stratify their patient population based on risk (age, sex, comorbidities) and assess their cost and utilization patterns to proactively address the needs of their most at-risk and rising-risk patients.
The organization lowered ER visits by 16%, admissions by 47%, and re-admissions by 40% for their patient population, which is below national averages. By using the MedeAnalytics augmented analytics solution for population risk stratification, the organization saw measurable impact to its bottom line.
Get our take on industry trends
Unfamiliar Healthcare Players, Interoperability and Social Determinants Round Out 2020 Megatrends
By Scott Hampel, president of MedeAnaltyics We conclude our 2020 Megatrends with an exploration of new players entering healthcare, the…
Read on...More Megatrends: Price Transparency, Telehealth, Individualized Medicine
By Scott Hampel, president of MedeAnalytics Now that we’ve dealt with Megatrends one through three, we’re approaching the next set.…
Read on...2020 Megatrends: Consumerism, Data Privacy and Security, AI
With 2020 two weeks old, it’s becoming clear the data produced in the healthcare industry by providers, consumers and payers will power and propel our 9 megatrends. Healthcare data is the foundation on which we’re building everything from healthcare outreach for the underserved to new Internet of Things-based healthcare programs to treatments designed just for you.
Read on...Why Unconventional Businesses Will Find Success in Healthcare: It’s the Data
It seems everyone is moving into healthcare. It’s a rapidly growing industry, historically dominated by large, well-embedded companies and organizations, and “pure tech” companies have had difficulty breaking in. That, however, is changing.
Read on...