By Kim Babberl
The amount of data produced daily has grown exponentially with nearly 90% of the world’s data generated in the last two years alone. To ensure we can make sense of this data, analysts must find meaningful ways to present the information to their audiences. They must do it quickly and concisely, all while making it seem effortless. And, it definitely is not.
Data visualization is a powerful healthcare tool because it can condense a lot of complicated information into a small space, allowing users to answer many questions at a glance. But that’s only possible when the visualization’s design allows those answers to show through. Pre-attentive attributes are visual properties that the human brain notices and processes in milliseconds before paying attention to anything else. Basically, it’s what you notice without having to make a conscious effort to notice. The chart below includes examples of pre-attentive attributes.
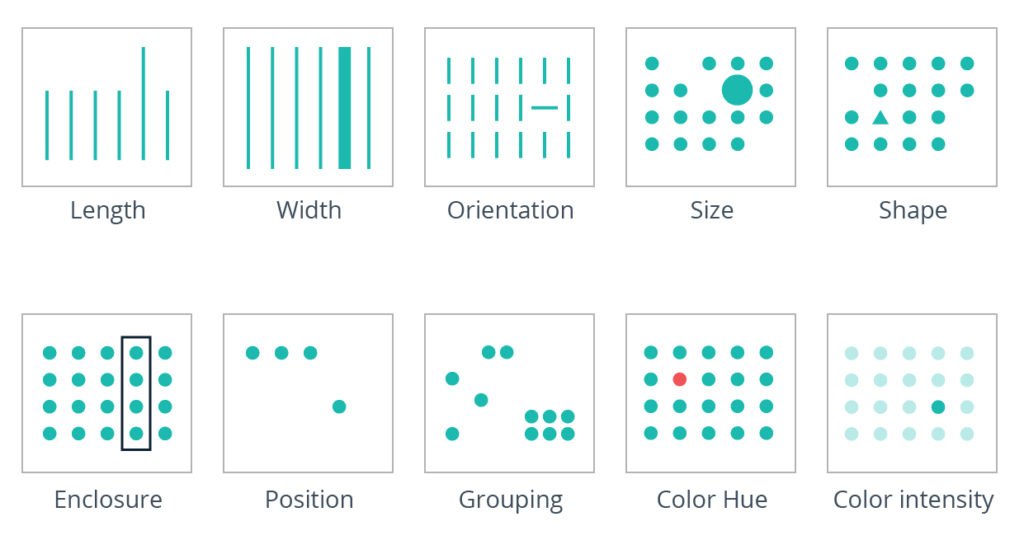
Let’s look at four of these attributes and how they can be leveraged as design building blocks to get the most out of your visual storytelling. Ready to dig into Grouping, Size, Position and Color? Read on!
Grouping
This is when underlying data is aggregated into individual data points, such as hourly vs. daily vs. weekly.
In data storytelling, it’s important to define the differences that are of interest to your audience. Reducing those differences to the minimal granularity needed will allow you to focus the design on the differences you actually care about and eliminate those you don’t.
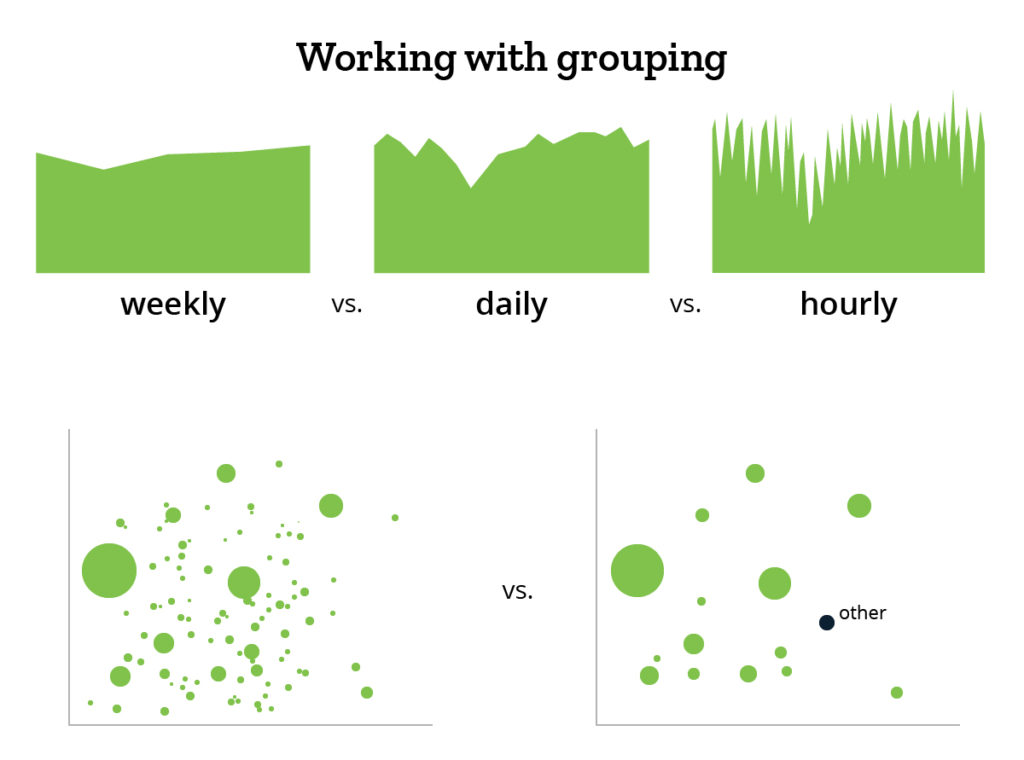
If you’re looking for patterns in utilization for a specific timeframe, say over seven days, you might not be interested in seeing dips over the weekend, which could be a normal business pattern. In this case, a weekly grouping will give you the insights you need without requiring a more granular, daily grouping.
Size
Should a data point be super-sized?
Simply put, how much visual mass does a given data point get? How many pixels? How much ink? Size as a visualization design element excels at representing quantities of measurement: people, dollars, orders, clicks and more. If you’re comparing metrics that are not quantitative, a different design element may be more helpful in distinguishing and comparing values.
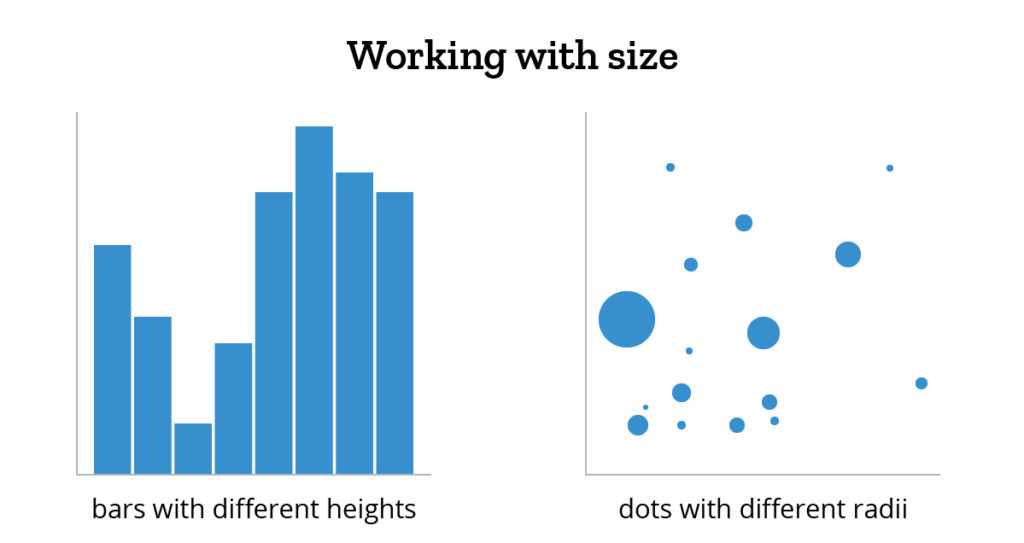
When working with size, it’s critical to maintain true proportions. If you change the y-axis of a bar chart to start at 100 instead of 0, you will interfere with the proportion of the bars. Essentially, a bar taking up twice as much space would no longer represent twice as much stuff. When using circles, you can’t just double the width to represent double the value, since it would actually quadruple the area of the circle. Instead, ensure the relative sizes of the data points preserve the true proportions of the values they represent so the user can accurately compare them.
Position
Where does data go?
Once you know which data to show and how small/big it should be represented, the next step is deciding where those data points should go. Positioning can be used in many ways. In a bar chart, bars can be placed in chronological order to represent time. In a scatterplot, the placement of a data point along the x- and y-axes can be useful in relating points to each other. The distance between data points is important: if two data points are near each other, we know they are similar in some way.
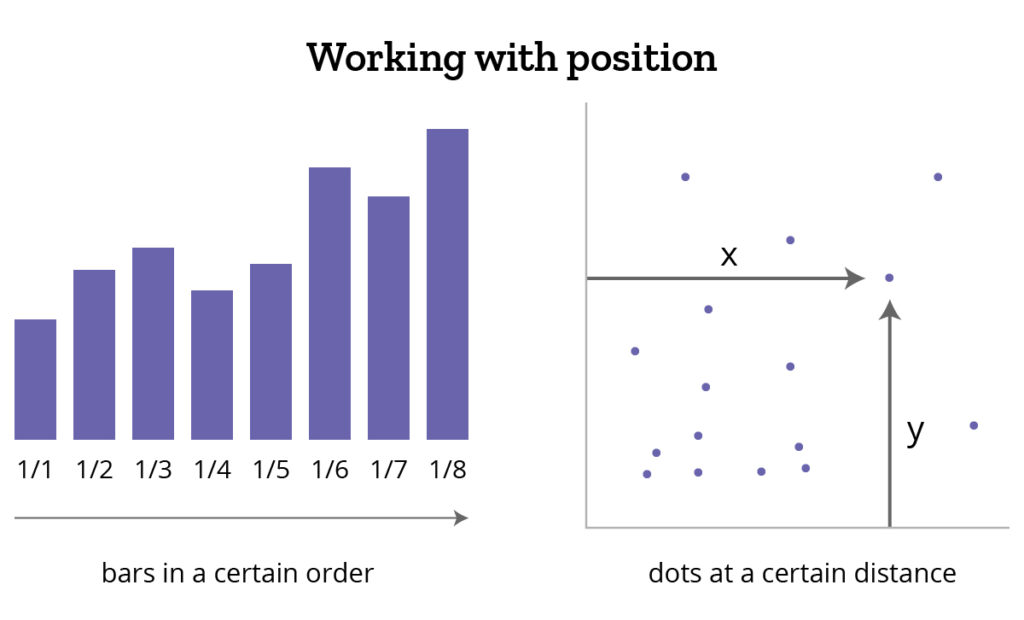
When creating a dashboard of various data charts/widgets/information, position is very important. Most users equate importance to the position on the dashboard with the top left corner being interpreted as most important. Thus, position isn’t just important within an individual data visualization but also encompasses the placement of a chart within a dashboard of information.
Color
Color emphasizes your point.
In many cases, the most obvious pre-attentive attribute in data visualizations is the use of color. Color may be used in one of three primary ways:
- Sequential
- Diverging
- Categorical
Sequential color is the use of a single color from light to dark. Population density may be effectively contrasted in this manner with more dense zip codes being darker than less populous areas. In the graph below, you can see comparative differences in unemployment rates.
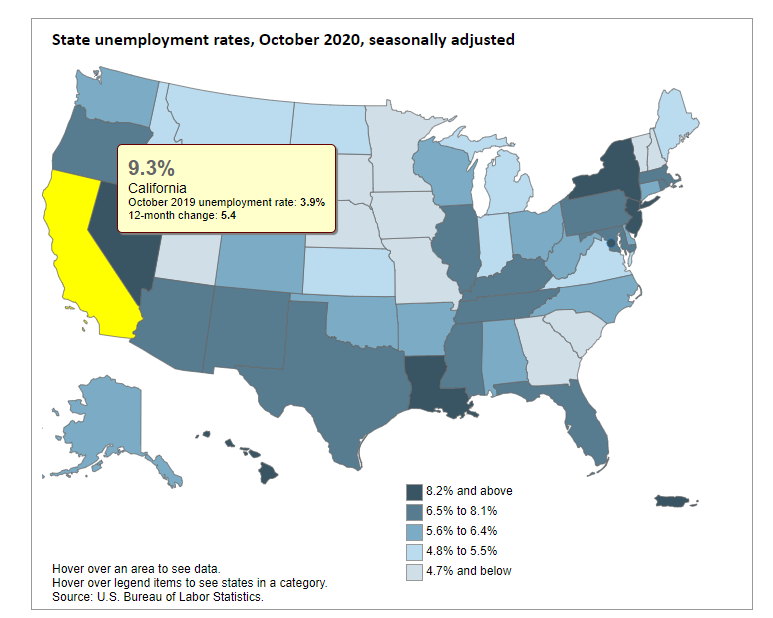
Source: https://www.bls.gov/charts/state-employment-and-unemployment/state-unemployment-rates-map.htm
Diverging color is used to show a range diverging from a midpoint, similar to sequential, but can be used to encode two different ranges such as positive and negative.
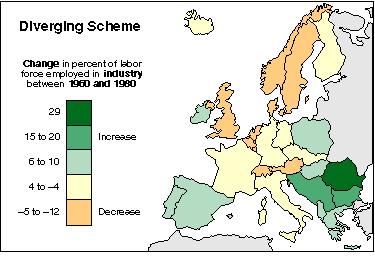
Source: https://web.natur.cuni.cz/~langhamr/lectures/vtfg1/mapinfo_2/barvy/colors.html
Categorical color uses different color hues to distinguish between different categories. Here’s an example that shows the most frequent service requests in NYC.
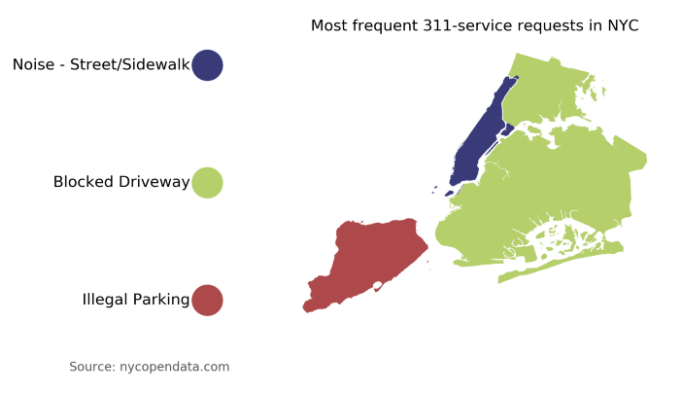
Source: https://towardsdatascience.com/create-categorical-choropleth-with-python-122da5ae6764
While color differences can be obvious to the user, there are a couple of things to keep in mind. Using color to represent gradation comes at the expense of interpreting specific values. Color is helpful and can be used as a grouping mechanism but is less effective in analyzing individual data points. Further, the user can’t perceive measurable differences in color patterns. When you see a darker shade of red above, it doesn’t come with the thought of “oh that’s about 20% darker.” Finally, as designers, we must always be cognizant of potential color blindness and ensure that data visualizations are universally readable.
Data visualization continues to be critically important in the world of analytics, especially in healthcare as data storytelling becomes more widespread throughout the industry. Following these best practices, while keeping important design elements in mind, will help ensure your story is heard, seen effectively and accurately, and most importantly understood.
So, happy visualizing! Let’s look at data in a whole new way!
Get our take on industry trends
Using data analytics to combat the maternal health crisis
With most pregnancy-related deaths being considered preventable, why is the United States facing a maternal health crisis? The country’s maternal mortality rate is the highest of any developed nation in the world and more than double the rate of peer countries.
Read on...Q&A with Dr. Lyle Berkowitz: Diving into the future of AI, analytics and precision medicine
Estimated reading time: 4 minutes After an excellent keynote session led by Dr. Lyle Berkowitz at our 2022 Impact Summit…
Read on...The missing piece to your Population Health strategy: A prescription for maximizing pharmacy data
The healthcare industry is swimming in data; sometimes organizations can even feel like they are drowning in available information. To…
Read on...Data, analytics and AI-enabled healthcare: Four key takeaways from the IS22 keynote
The 2022 Impact Summit (IS22) was outstanding for a plethora of reasons—and Dr. Lyle Berkowitz is foremost among them. For this year’s keynote, Lyle…
Read on...